Credit: New Africa / Shutterstock
The AI Playbook: 6 steps for launching predictive AI projects
By
Companies are hankering for predictive analytics that promise to boost sales, cut costs, prevent fraud, and streamline operations.
Yet most organizations are failing to achieve their desired outcomes. An MIT Sloan Management Review and Boston Consulting Group study found that just 10% of companies garnered significant financial benefit from their AI investments. And in a survey by Rexer Analytics, just 22% of data scientists said that their new initiatives are usually deployed and operationalized across the enterprise.
Many predictive machine learning projects fail because they focus too much on technology alone as opposed to advancing technology as a strategic business project, according to Eric Siegel, a consultant and former professor at Columbia University and the University of Virginia.
In his new book, “The AI Playbook: Mastering the Rare Art of Machine Learning Deployment,” Siegel makes the case that organizations are not seeing value from AI because they lack an effective business paradigm for running machine learning projects. Because most machine learning projects are highly technical, they often fall under the domain of experienced data science professionals. The result is a disconnect between the data experts preparing data and developing and operating AI models and the business stakeholders in charge of running large-scale operations who stand to benefit from predictive insights.
“By focusing so much on the modeling science rather than its deployment, it’s like being more excited about rocket science than the actual launch of the rocket,” Siegel said in a recent MIT Sloan Management Review webinar. “That’s where we are today.”
To boost success, Siegel said, businesses need a standardized playbook for machine learning projects that is accessible to business professionals and can help them participate in the life cycle of predictive analytics projects.
Otherwise, “both sides point to the other and say, ‘Running and managing this business-level process is not my job,’” he explains. “It rests in no-man’s-land, and that is the last remaining ingredient before we get more wide-scale success and deployment.”
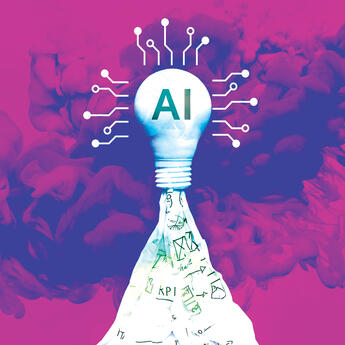
AI Executive Academy
In person at MIT Sloan
Register Now
6 steps for launching machine learning projects
To bridge the divide, Siegel advocates for something he calls “BizML,” a set of business practices for running predictive machine learning projects.
He outlined six steps to foster collaboration among business and technical stakeholders throughout all phases of machine learning deployment:
Establish the deployment goal. To derive any real value from machine learning, businesses need a defined value proposition that details how the technology will impact operations. Data scientists can’t do this in a vacuum. It’s important that business stakeholders who are intimately familiar with the pain points and opportunities are technologically savvy enough to participate in realistic goal setting.
Establish the prediction goal. While modeling and prediction involve complex mathematics, business goals need to be kept in mind. Business users need to have a semitechnical understanding of the technology so they can share their specific domain knowledge while also defining what the machine learning model is intended to predict for each use case.
Establish the right metrics. Determine the salient benchmarks to track during both model training and model deployment. In addition, identify what performance levels must be achieved for the machine learning project to be considered a success. Typically, most machine learning projects are grounded by technical metrics such as precision, recall, or accuracy. Organizations need to shift their focus to business metrics like profit, ROI, savings, and customer acquisition, Siegel said.
Prepare the data. Define what the training data should look like, and ensure that data is in the desired format. This critical step is nonnegotiable because it is the linchpin to experiencing high-value results, Siegel said.
Train the model. Next, the prepared data is used to train and generate a predictive model. Data experts lead the charge here, but there is always room for additional business input.
Related Articles
Deploy the model. Use the model to render predictive scores and in turn, use those scores to improve business operations. It’s also important to maintain the models through ongoing monitoring and periodic refreshing.
While the last three steps are more technical than the first three, all of them require deep collaboration between technology and business stakeholders. Building bridges to connect the two camps requires investment and engagement in the right change management practices to ensure adequate understanding of machine learning across stakeholders throughout the business.
“Change management challenges aren’t new in general, but when it comes to machine learning projects, the need to shrewdly manage change is often overlooked,” Siegel said. “Machine learning delivers a rocket, but those in charge must still command its launch.”
Watch the webinar: How to Succeed with Predictive AI