Credit: TippaPatt / Shutterstock
Generative AI as a new platform for applications development
By
Personal computers, smartphones, and cloud services have spawned entire ecosystems that advance innovation.
Now there are signs that generative AI is poised to pick up that mantle, serving as a foundational platform that enables a wide variety of software applications and services, according to a paper by MIT professors and As with past market-disrupting technologies, generative AI is starting to reap the benefits of an evolving ecosystem of infrastructure layers and enabling tools and frameworks, along with a rapidly growing set of applications.
While the pieces are still coming together, that confluence sets the stage for generative AI to become a powerful enabling technology, Cusumano said, and software developers and technology producers need to learn how to train systems and embed generative AI in applications, if they haven’t already.
“Generative AI is the next chapter — it’s the innovation platform for the modern age,” Cusumano said. “But we are still early on.”
The flurry of generative AI ecosystem activity isn’t lost on industry watchers, who expect the emerging technology to have a sweeping impact on the global economy. Goldman Sachs is projecting that generative AI could lead to a 7% increase in gross domestic product, with productivity growth lifted by 1.5 percentage points over a 10-year period.
A generative AI ecosystem begins to evolve
As with previous innovation platforms, generative AI’s momentum is being fueled by a multilayered ecosystem. Infrastructure providers like Nvidia produce graphics processing units and other hardware while others offer cloud services for running generative AI software. Foundational models, or large language models, are produced by major technology providers, like OpenAI, Meta, and Google, as well as by smaller, specialized firms. Applications, now numbering in the hundreds and growing at pace, are continuously being released by small and well-known players — both vertical solutions targeting specific industries and horizontal products aimed at a broader audience.
Past innovation platforms, like PC operating systems and online transaction marketplaces, also benefited from major-player participation, according to the researchers. In addition, they gained traction through the phenomenon known as “network effects,” which is starting to catalyze for generative AI. Network effects come into play when more users attract third-party applications, which in turn attract more users and more applications, with the cycle repeating to drive exponential growth.
“We are sure of one thing: Now is the time for companies, universities, governments, and technology experts to think carefully and to think together,” the researchers write. “We all need to understand better the costs, benefits, trade-offs, and potential dangers of GenAI as a new applications platform and transformative technology that is already shaping our common future.”
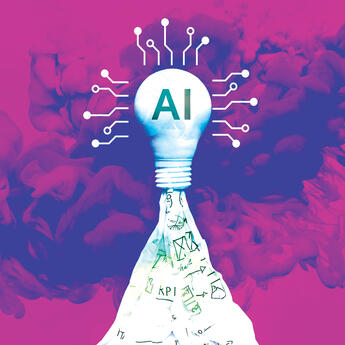
AI Executive Academy
In person at MIT Sloan
Register Now
Challenges on the horizon
As they move forward, organizations need to consider the following challenges identified by the researchers:
Concentration of market power. The downside of powerful network effects is diminished competition. Software developers tend to coalesce around the most popular or accessible LLMs to build applications. In addition, only a portion of companies will have the money to develop and train foundational models. These dynamics are likely to ensure the continued dominance of technology giants like Google, Meta, and partners Microsoft and OpenAI, the researchers write. Large players could drive out startups and open-source providers from the generative AI ecosystem. “Dominant platforms can stifle innovation, harm users and competitors through predatory pricing, and produce lasting monopoly profits for a small number of firms,” the researchers write.
Some trends — such as the adoption of standard interfaces to popular LLMs — are helping to minimize lock-in for developers. Additionally, “startups such as DeepSeek from China, with its open-source large language model, may alter the balance of power away from the Big Tech firms in the United States and make running generative AI software cheaper,” Cusumano said.
Data ownership and privacy. Data privacy, bias, and content ownership issues that surfaced with earlier innovation platforms also apply to generative AI. Ongoing litigation — for example, The New York Times’ lawsuit against OpenAI and Microsoft for copyright infringement after they used news articles to train ChatGPT and Microsoft Copilot — have not yet been resolved, leaving questions about the fair use of published content as training data.
Hallucinations and information accuracy. LLM responses can be irrelevant, be factually wrong, or contain harmful content. Reliability can be improved through use of external tools, and accuracy tends to improve as an LLM increases in size. Nevertheless, this ongoing challenge requires developers and users to put robust mechanisms in place to check LLM output and correct errors. Among the options noted by the researchers: keeping humans in the loop throughout the process and investing in tools designed to detect instances of fake text, audio, or video.
Regulation versus self-regulation. Most complex, emerging technologies require some combination of self-regulation and government regulation. The researchers make the case for a mix of government and private sector oversight, especially as tech giants continue to dominate generative AI technologies. “Checks and balances need to be in place to mitigate the risk of regulatory capture by well-funded LLM providers,” the researchers write.
Related Articles
Economic and social disruptions. Generative AI has the potential to upend existing hierarchies and company stature in entire industries, specifically high tech. At the same time, a broader disruption is likely to hit white-collar jobs, with the technology impacting employment status as well as the nature of the work. People in many occupations — among them teachers, journalists, lawyers, stock traders, computer programmers, and corporate planners — may find their jobs made obsolete, enhanced, or vastly altered.
Environmental impact. The computing resources required for LLM training and responses is huge. While work is underway to make LLMs and resulting applications more energy efficient, companies and governments need to closely evaluate the trade-offs between generative AI’s energy use and benefits.
Unintended consequences
The researchers caution that there is no crystal ball to predict where generative AI technology will go. While this new platform for application development will create opportunities for automation and bring efficiencies to many jobs, there is real potential for personnel reductions and significant changes in work processes. The possibility for inappropriate generative AI use in high-stakes scenarios could also be catastrophic.
With all of that in mind, it is crucial that organizations achieve a better understanding of the costs, benefits, trade-offs, and potential dangers of generative AI as a new application platform and transformative technology that is already shaping our common future. “This technology is going to be in everything,” Cusumano said. “It is pervasive and we’re going to get used to it.”
Read the paper: Generative AI as a platform for applications development