MIT Sloan Health Systems Initiative
Research Spotlight: Novel, Robust AI-Based COVID-19 Model Predicts Disease Prevalence and New Infections
The past may not be a direct indicator of future performance. However, with nationwide, state-by-state case data from a variety of sources, the research team led by Professor Georgia Perakis developed a model that predicts disease prevalence weeks in advance based on cases and deaths at the state level. The engine underlying their work combines machine learning and epidemiology to develop a novel, three-part, weighted algorithm. Professor Perakis’ team is composed of five PhD students from the Operations Research Center: Mohammed Amine Bennouna, Divya Singhvi, Omar Skali Lami, Ioannis Spantidakis and Leann Thayaparan as well as an Executive MBA student, Boyan N. Peshlov. David Nze Ndong, a student from the Master of Finance Program, joined the team recently.
Predicting COVID-19 Testing Results and Number of Deaths
They are predicting the number of COVID-19 cases in each state and in Washington, D.C. by searching for similar patterns in the past from others states. Then, they use a weighted combination of several types of models to make the prediction. Their work is at the intersection of public health and operations research: they use epidemiological models as well as machine learning algorithms. The epidemiological models help them calculate the overall prediction; then machine learning adeptly deployed allows them to account for additional state-level characteristics. Specifically, the team used COVID-19 testing data from March 15 through June 15 to predict the results for the end of June and July. For most states, the model came very close to the actual number; in others the model will be further adjusted. The model can be adjusted in two ways: with additional data and by further refinement to the model’s structure to account for the specific intricacies of the COVID-19 pandemic that are surfacing as the pandemic unfolds. The model already accounts for changes in behavior of the US population through their mobility data, but further refinements include adding details such as explicitly accounting for government policies (e.g., pushes for mask wearing and social distancing), and by learning from systemic changes like second waves. At first the model was able to predict positive test result totals within 6% of the actual number in the country. With further refinement, as of the middle of June, the model was able to predict the actual number of positive tests within 2.2% and the actual number of deaths within 3.5%, two weeks in advance. Perakis and team’s next step is to refine this model from nation-wide and state-level numbers to county-level predictions. This information may be key to informing policy decisions, such as when and how to open universities and large businesses.
Predicting Prevalence - How Many People Are Infected?
The model extends beyond the predicting case totals based on COVID-19 tests. While this number allows the researchers to test the accuracy of their model by comparing how well their model matches the testing data, the true number of people with COVID-19, the prevalence, is greater than the number of people who test positive, since not everybody is tested.
A separate part of the project addresses this challenge. The team extrapolates from the model and uses probability theory to allow the algorithm to calculate the true prevalence, the actual number of people infected with COVID-19. This number includes people who are displaying symptoms, those who are pre-symptomatic and those who are asymptomatic. The goal is to account to everyone who is infectious. For those working to make decisions using the best public health data, this information is crucial.
Perakis’ leadership and her team’s efforts are an example of the timely, innovative and valuable work taking place at MIT Sloan. Machine-learning-based predictive models adroitly adapted to public health problems provide timely and actionable results that may not be otherwise available.
Featured Researcher
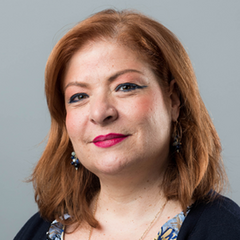
Georgia Perakis
Management Science
John C Head III Dean (Interim), MIT Sloan School of Management
Learn More