Credit: jpkirakun / iStock
Artificial intelligence is getting all the buzz in the business world. But people often talk about “doing” AI without realizing that it’s just a tool for getting things done — a costly and complex tool.
“Like any tool, it creates no value unless it’s used properly,” said a principal research scientist at the MIT Center for Information Systems Research. “AI is advanced data science, and you need to have the right capabilities in order to work with it and manage it properly.”
In a new research briefing, Wixom and CISR research fellow Cynthia M. Beath outline three principles for implementing AI projects: Build the right capabilities, involve stakeholders from across the organization, and focus on realizing value.
An important theme linking the three principles is data monetization, which is also the topic of the recent book “Data Is Everybody’s Business,” by Wixom, Beath, and Leslie Owens.
AI requires “supercharged” data monetization
In simple terms, data monetization is converting data into financial returns. Datasets could be sold to third parties, studied to improve processes, or used to create new business opportunities.
Seeing a return on investment from AI requires a “supercharged” data monetization strategy, Wixom said. There’s significant cost associated with the infrastructure, capabilities, and talent needed to get AI initiatives off the ground. And any enterprise that builds AI into a product — even if it’s something relatively simple, such as a customer service chatbot — must bear the associated legal and ethical responsibilities.
“So many missteps can occur,” Wixom said. “What we care about in the AI conversation is helping organizations embrace AI technology in a way that improves financial health.”
For example, many knowledge workers are using generative AI tools, such as ChatGPT, to write emails, create presentations, or pull key takeaways from meeting transcripts.
These conveniences shouldn’t be conflated with value, Wixom said. “If you invest millions of dollars in the capability to use AI in a sustaining way, is email productivity going to drive millions of dollars in value?” Wixom asked. “You need to know what your company needs.”
The CISR research briefing includes the example of CarMax, which used ChatGPT to aggregate data from customer reviews and other information sources to write summaries for 50,000 used cars listed on its website. A task that would have taken years to complete and required hundreds of content writers was accomplished in a matter of hours, the company’s chief information and technology officer said last year.
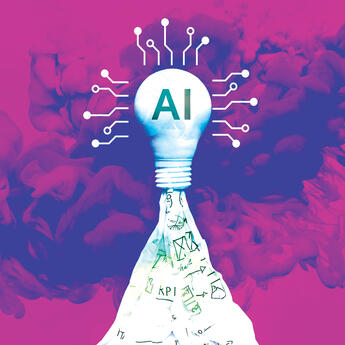
AI Executive Academy
In person at MIT Sloan
Register Now
Below are more details on the researchers’ three principles aimed at guiding enterprise AI investments that generate value:
1. Invest in practices that build AI capabilities.
Successful AI use depends on advanced capabilities in data science, data management, data platforms, and acceptable data use.
Organizations may be tempted to forgo these investments if they purchase an off-the-shelf AI tool or have one custom-built for them. However, Wixom said it’s even more important for these organizations to build these capabilities to avoid running into trouble downstream.
“You have to understand the technology enough to trust it,” she said. “You don’t have to know the exact way neural networks work, but you do need to know how data scientists validate them — and you need to know what you don’t know.”
2. Involve all stakeholders in the AI journey.
Roles and titles differ among organizations, but the people who use AI tools, develop products, or offer solutions should be considered key stakeholders. Involving a variety of stakeholders in initiatives can help those without a background in data science better understand what AI can do, how much it costs, and how long it takes.
The CISR research briefing looked at the Australian Taxation Office, where business users provided regular feedback to data scientists about how AI models were performing. This helped all stakeholders understand how AI models made decisions, and it ensured that models had more positive benefits and fewer negative consequences. After implementing the models, which predicted tax-filing behaviors and offered nudges to citizens who needed to update work-related claims, the agency saw 113 million Australian dollars ($74 million) in changed claim amounts.
3. Focus on realizing value from AI.
In “Data is Everybody’s Business,” Wixom and her co-authors spell out five steps in the value creation cycle. Wixom explains them in the context of a common example in healthcare: predicting which patients in a hospital are at high risk of falling, and intervening to prevent it from happening.
- Data. This comes from multiple sources, such as a medical history from electronic health records and current data from medical devices at the bedside.
- Insight. This is where AI enters the picture to analyze data assets and predict who faces a high risk of a fall. Preventable falls are frequently linked to worse outcomes and higher costs of care.
- Action. Here, insights inform changes to best practices. For example, a hospital may update its policies and send alerts to the nearest nurse when an at-risk patient starts to move in the bed — a sign that they may be trying to get up.
- Value creation. In this scenario, the new policies result in fewer preventable falls, increased patient satisfaction, and shorter patient stays.
- Value monetization. This occurs when the changes can be linked to tangible value creation. It may manifest as a reduced cost of care, which benefits hospitals compensated under performance-based contracts. It could also mean that fewer staff members are needed in a given unit.
A tough road from creation to monetization
Related Articles
Organizations need to plan out the five steps of value creation before any AI project commences, Wixom said. That will help executives see exactly what it will take to achieve returns on investment.
Monetization is the most difficult step. Many organizations get to value creation but are halted there. In a hospital, for example, additional revenue or reduced staffing costs may never materialize.
“You have to find a way to pay for this,” Wixom said. “Otherwise, you can’t sustain the investments, and then you have to pull the plug.”
Yet all isn’t lost if there are meaningful outcomes, such as a reduction in preventable falls, that don’t achieve monetization. In most cases, an organization now has the building blocks — data, insight, and action — to take on additional AI projects down the road. And it has experience successfully selling AI to stakeholders by focusing on what it can get done.
But without monetization, “AI will end up being a tool that sits on a shelf,” Wixom said. “You need to be talking about AI along with specific initiatives and the outcomes you want to achieve using it. And those outcomes need to be compelling for your organization.”