Credit: Mimi Phan / Kitawit Jitaton / Shutterstock
For the last few years, companies have been experimenting with use cases for generative artificial intelligence. At least one common theme is emerging: Generative AI, paired with data analytics, helps companies execute projects and solutions with speed.
This is the most significant trend uncovered from the 2024 MIT Sloan Master of Business Analytics Capstone Project program, which partners students with companies that are looking to solve a business problem with data analytics. The program also provides a look at what problems were top-of-mind for organizations in the beginning of 2024. This year, one theme among the 41 projects was that companies are looking to take advantage of generative AI.
To contrast generative AI and traditional AI, students are starting to use the term “legacy machine learning,” according to MIT Sloan lecturer who leads the Capstone program. “Legacy machine learning approaches enable precision — algorithms identify consumer preferences to the individual level, identify an individual’s likelihood of voting, or prescribe treatment to individual patients. Generative AI unlocks speed.”
All these projects reached the level of a functioning, in-use product and were tested with live data in some capacity, Levine said. About half are live and actively supported by the companies.
Here’s a look at the problems businesses wanted to solve and how they are pairing generative AI and analytics for novel business solutions.
Speed to market
Two life science companies looked to use generative AI and large language models to increase speed to market for drugs.
Biopharmaceutical company Pfizer aimed to reduce the time required to get new drugs to market. One way was to speed up the knowledge transfer process, which is when relevant research and development and other documents are transferred to the manufacturing department. Using manual methods, it could take nine months per molecule to classify thousands of documents for a drug, and observations can be lost, hidden, or siloed off from the broader enterprise. The MIT Sloan team used more than 33,000 documents to build a suite of products that makes scientists’ work easily available by quickly recognizing and retrieving information. This is already accelerating the speed of discovery and the speed to market for therapies.
Leaders at biopharmaceutical company Takeda wanted to know whether the company’s clinical trials included more or longer visits, more procedures, or more invasive procedures compared to clinical trials led by peer companies. The MIT Sloan team used data on more than 500,000 clinical trials from a government website to establish benchmarks for clinical trial participants’ visit frequency, length, and numbers of procedures. Combining this analysis with generative AI, the company can now design clinical trials more quickly, which will also speed up critical drug development timelines.
Quicker responses to customers
Three companies are demonstrating the ability to pair data with generative AI to more quickly deliver real-time insights and guidance to customers.
Insurance company CogniSure aimed to extract important information from thousands of PDFs and emails submitted by customers, while retaining accuracy. This is especially difficult when information is presented in different forms, such as text fields and check boxes. The MIT Sloan team used generative AI to develop a method for quickly reading any file, which will help the company deliver insurance quotes more quickly to people looking for a new policy.
Given that calls can cement a company’s relationship with a customer — or result in lost business — telecommunications company Comcast wanted to improve its real-time response to nearly 6 million calls per month. The MIT Sloan team used a combination of customer data, data from an interactive voice response system, historic transcripts of calls, and seven-day churn results to build a framework that improves agent response to a customer during a call. This allows agents to quickly identify high-risk customers and identify targeted follow-up calls. It also provides insights about trends to management.
Global shipping and transportation company CMA CGM traditionally relied on its trade team’s experience to make pricing decisions. The MIT Sloan team created a new generative AI system built on more than 70 million rows of transactional data that can provide more immediate predictions and guidance on dynamic pricing and the likely effects of price changes. These insights are available to agents through a dynamic pricing advice assistant, allowing them to give quicker, more accurate information to customers.
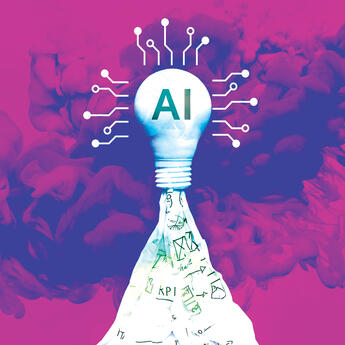
AI Executive Academy
In person at MIT Sloan
Register Now
Speedier communication, both internally and externally
Two other companies are using generative AI to quickly publish customer communication and more easily share information within the company.
Retail store Dick’s Sporting Goods sends one to two emails per day to customers on their subscription list. Previously, the emails were created manually and had a limited amount of personalization. To create more personalized campaigns more quickly, the MIT Sloan team used generative AI paired with demographic and loyalty data, email templates, email interactions, and transaction history to create personalized email templates. The result is improved click-through rates per email and reduced time required for marketing campaign preparation.
At consulting firm McKinsey & Co., employees share knowledge and findings with their colleagues in a database, using tags to identify documents related to specific fields. In the past, this information has been manually curated and tagged, which was time consuming and only about 50% accurate. A new generative AI tool created by the MIT Sloan students can label 26,000 documents annually, ensuring that the information is available at the right place and time.
Beyond speed: managing accuracy, generative AI, and the workforce
While there are many benefits to using generative AI to quickly respond to customers, develop drugs, or communicate with important stakeholders, accuracy is also important, Levine noted, and each team assessed the accuracy of their projects with various methods.
It’s also important to understand how using generative AI will affect workers. Professional services company Accenture looked to help its clients redesign their workforces and adopt effective generative AI strategies. The MIT Sloan team analyzed task and skills data for 851 jobs, 332 tasks, and 7,748 skills; job transition data, which includes wages, education and experience; and employment statistics across enterprises. The result is a tool that predicts the effect of generative AI on large enterprise work forces, which will help companies deploy technology strategies and reskilling initiatives.