Credit: Alfonso Soler / KrulUA / iStock
Artificial intelligence technology is evolving quickly, and most organizations are struggling to keep up. Experimentation and pilot projects are well underway as companies try to create a framework for capitalizing on AI.
To achieve desired outcomes, leaders need to be equipped to lead AI initiatives, according to MIT Sloan senior lecturer the faculty director of a new MIT Sloan Executive Education course about leading AI-driven organizations. The course looks at how leaders can apply AI technologies to optimize value in business operations, guide organizations beyond exploration to implementation, and ensure the responsible application of AI, which is crucial as expanded use cases increase enterprise risk.
“There’s currently a real delta between the numerous proofs of concept and full-blown AI strategies,” McDonagh-Smith said. To close that gap, leaders need to create a plan for using AI that takes into account key business priorities, the organization’s data strategy, and employee skills.
Throughout, leaders need to remember that the key to AI is “not humans plus machines, but humans multiplied by machines, augmenting and assisting our capabilities across the existing and emergent tasks performed in our organizations,” he said.
McDonagh-Smith had the following advice for leaders who are guiding AI initiatives:
Create an AI playbook
There is no singular route to large-scale AI deployment. Rather, McDonagh-Smith advises leaders to use their answers to these three sets of questions to map out their organization’s journey:
What are the organization’s critical business problems and strategies, and can AI be used to deliver upon on those strategies?
AI use isn’t about staying ahead of the curve or being part of a trend. Organizations need to clearly define the business problem they are trying to solve and fully understand the breadth of available AI technologies and techniques available to them so they can match a solution to the problem. One way to facilitate this exercise is to deconstruct a business challenge into a set of subproblems. “In the field of AI, you determine which technologies and techniques are going to be helpful for these subproblems,” McDonagh-Smith said. “You also need to abstract and separate the signal from the noise.”
Is the organization’s data AI-ready (that is, are the datasets that are being trained suitably complete and managed with the proper governance)?
A key ingredient to successful AI is having the right datasets for exploring, analyzing, and recognizing patterns for each use case and business problem. Organizations need to elevate their data game by launching data cleansing and data management initiatives, establishing data governance, and aligning with the right third-party data partners where and when it makes sense.
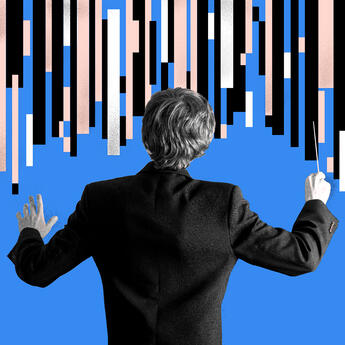
Leading the AI-Driven Organization
In person at MIT Sloan
Register Now
What is the AI maturity level of organization employees, and where are there key skills gaps? What kind of programs should be in place to upskill employees on critical new competencies?
AI skills are not overly abundant, so organizations need programs to upskill and train employees on technical skills and technical decision-making to fully leverage AI’s capabilities.
Culture is a big part of the equation: Organizations will need to create silo-busting cross-functional teams, make failure permissible to encourage creativity, and encourage innovative ways to combine human and machine capabilities in complementary systems.
Given the current frenzy around AI, level-setting expectations is a crucial element of enterprise-scale AI success. One way to achieve this is to recognize the key duality of AI: —Organizations need to adopt a fast-and-slow, two-tier approach to their enterprise AI strategies, McDonagh-Smith said. “Fast experiments and proofs of concept are fed into the creation of slower, longer-term strategy,” he said, pointing to the idea about two modes of thinking — one fast and intuitive and the other slow and analytical — that Nobel Prize-winning behavioral economist Daniel Kahneman examined in his work.
“By applying Kahneman’s ‘thinking fast and slow’ [concept] to how we use AI today, we can enhance organizational capabilities and competitiveness tomorrow,” McDonagh-Smith said. In addition, “we’ve got to be careful that the enthusiasm and optimism doesn’t get waylaid and that we’re able to have a practical, reasonable strategy for how we’re going to integrate AI into our businesses.”
Embrace experimentation and responsible AI
With those questions answered, organizations have a strong foundation for moving forward with AI initiatives. When doing so, McDonagh-Smith said, leaders should remember the following:
Related Articles
Embrace experimentation while setting strategy. With AI, organizations need to continuously experiment, build proofs of concept, and promote sandbox activities. At the same time, they need to capture the lessons and data from these experiments — both successes and failures — and use them to inform medium-to-longer-term AI strategies. Most organizations lean too far in one direction or the other, McDonagh-Smith said.
“We might be experimenting but not envisioning what this means for a medium-to-longer-term strategy,” he said. “But if all we’re thinking about is medium-to-longer-term enterprise strategies, we’re never going to be able to fine tune enough of the proofs of concept to create value for our businesses.”
Ensure responsible AI use. Without the proper safeguards, AI opens the door to significant enterprise risks, including potential brand damage, privacy infractions, and the spread of dangerous misinformation. Companies need to identify and communicate their ethical values, create accountability through organizational structures, and take continuous steps to detect and remediate bias.
Watch: LinkedIn Live session about driving transformation with AI
Read next: How continuous learning keeps leaders relevant in the age of AI